Digital Trust Ecosystems
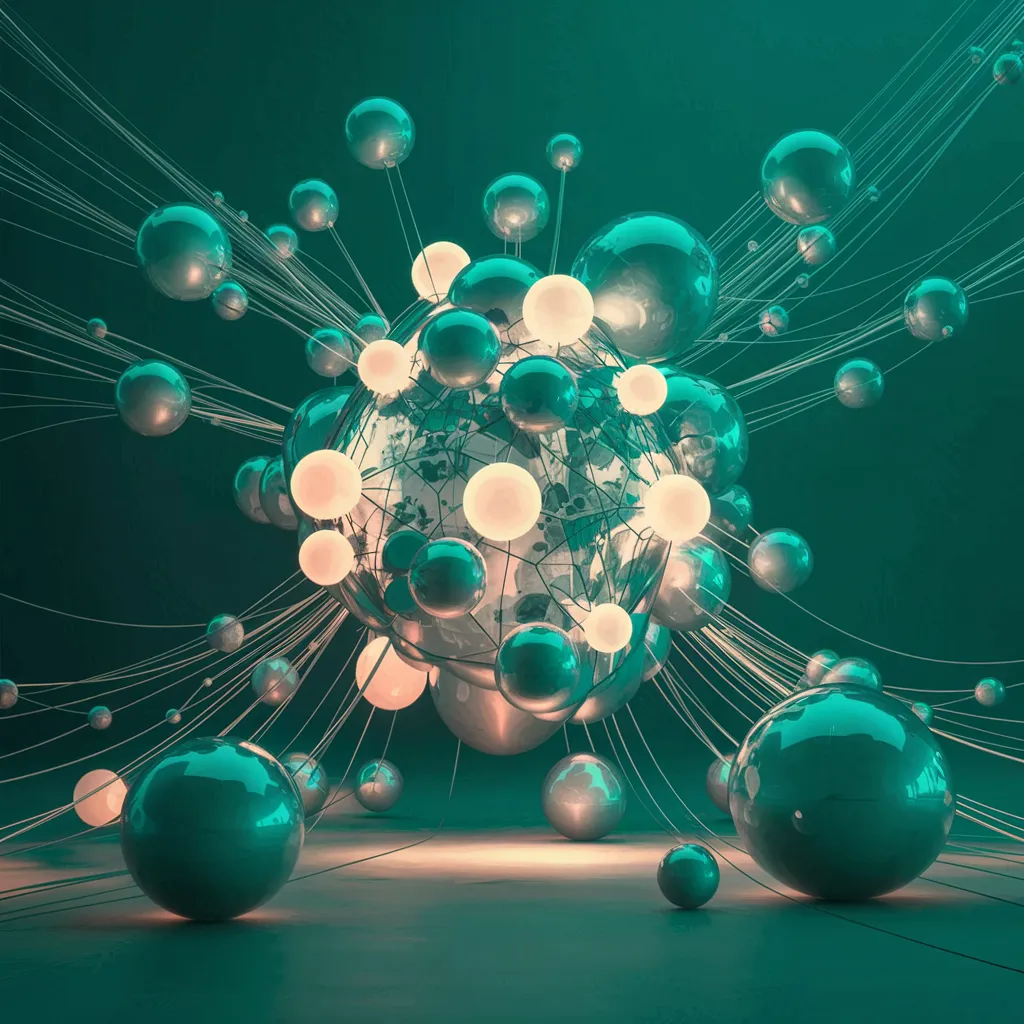
The true value of digital trust technology is realized at scale when users are able to leverage their credential for a large variety of use cases.
Successful and sustainable digital trust adoption requires an ecosystem approach, where use cases are explored in context to their environment (e.g. service area, sector). Beginning with a pilot and a small user group, initial efforts can be scaled up by equipping more users with corresponding credentials and exploring additional use cases.
Roles in a Digital Trust Ecosystem
Actors in digital trust ecosystem usually take up at least one of the following roles:
- Issuer: An issuer defines and produces a digital credential and makes it available to a holder.
- Holder: A holder receives a digital credential from an issuer and presents it to a verifier.
- Verifier: A verifier trusts in the issuer and grants the holder access to their service.
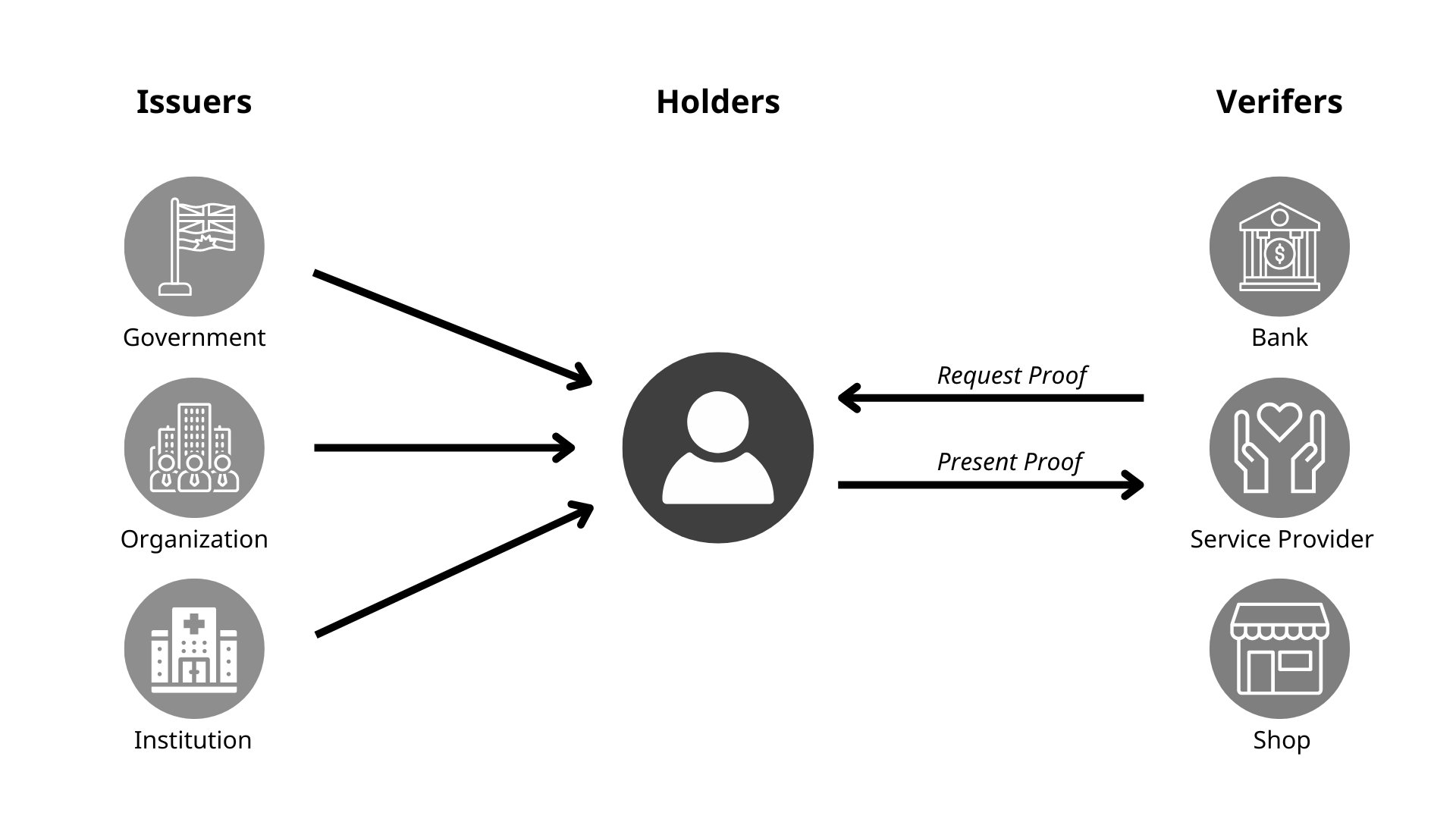
The roles within the digital trust ecosystem—issuer, holder and verifier—are not rigid. They are fluid, meaning they can be adopted by a variety of actors, including individuals, government entities and businesses, depending on the context of the interaction.
Digital Trust in the Justice Sector
Visualizing the relationships and interactions in a digital trust ecosystem can help to understand and apply the ecosystem approach.
The graphic below shows what the current digital trust ecosystem in the justice sector of British Columbia looks like.
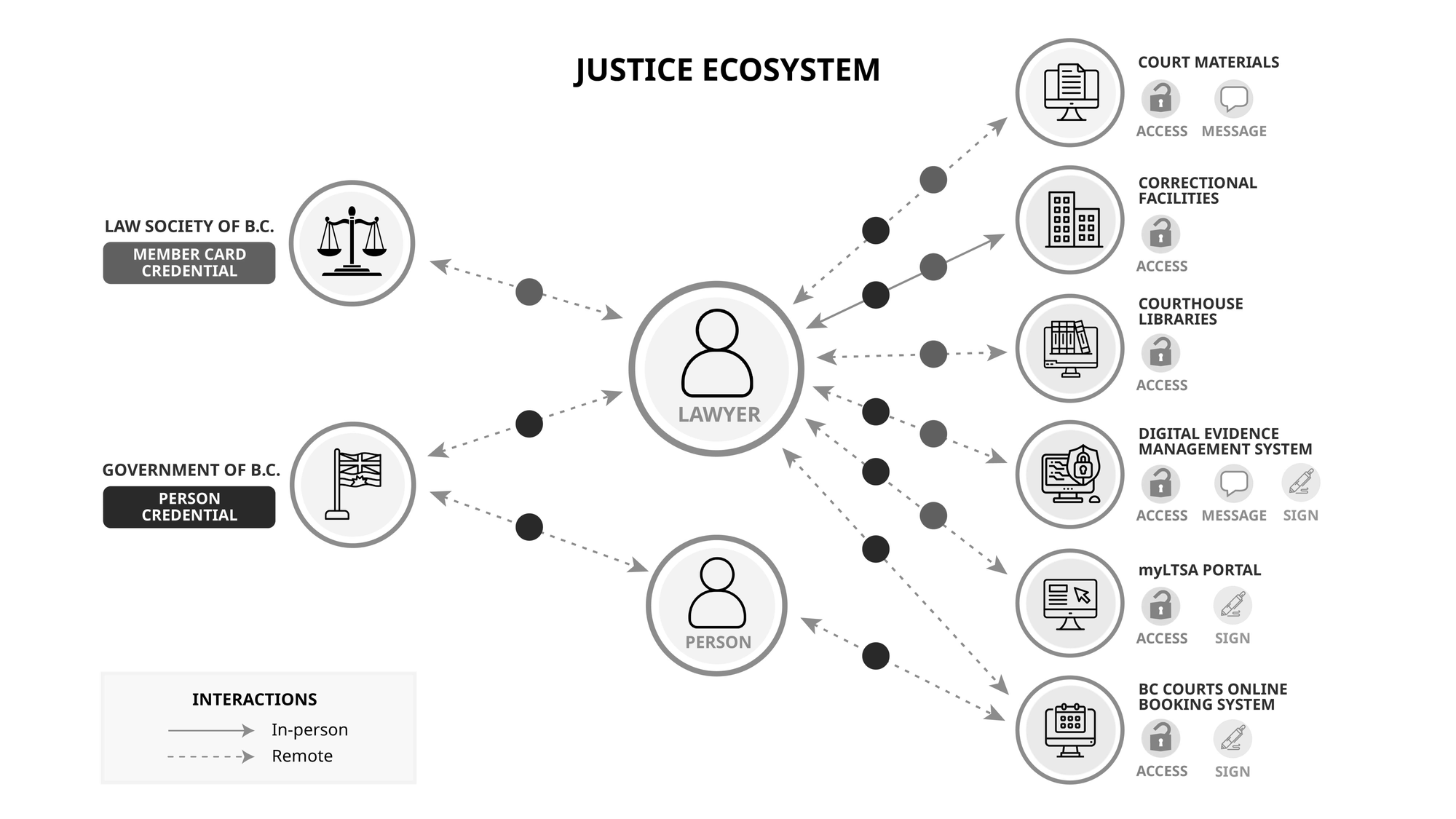
In the justice ecosystem graphic above, the roles are distributed as followers:
- Left side: The Law Society of BC and Government of B.C. are issuers.
- Middle: The lawyer and person are holders.
- Right side: The entities managing the services behind each of the use cases are verifiers.
Digital Trust in the Health Sector
In Abramson et al. we present a decentralized trust architecture tailored for federated learning (FL) in healthcare settings. By employing Decentralized Identifiers and Verifiable Credentials, the framework ensures that only authenticated entities—such as hospitals and researchers—participate in the FL process, thereby mitigating risks associated with data poisoning and model theft. We demonstrated a proof of concept using Hyperledger Aries, establishing secure, peer-to-peer communication channels that facilitate collaborative machine learning on sensitive mental health data without compromising patient privacy.
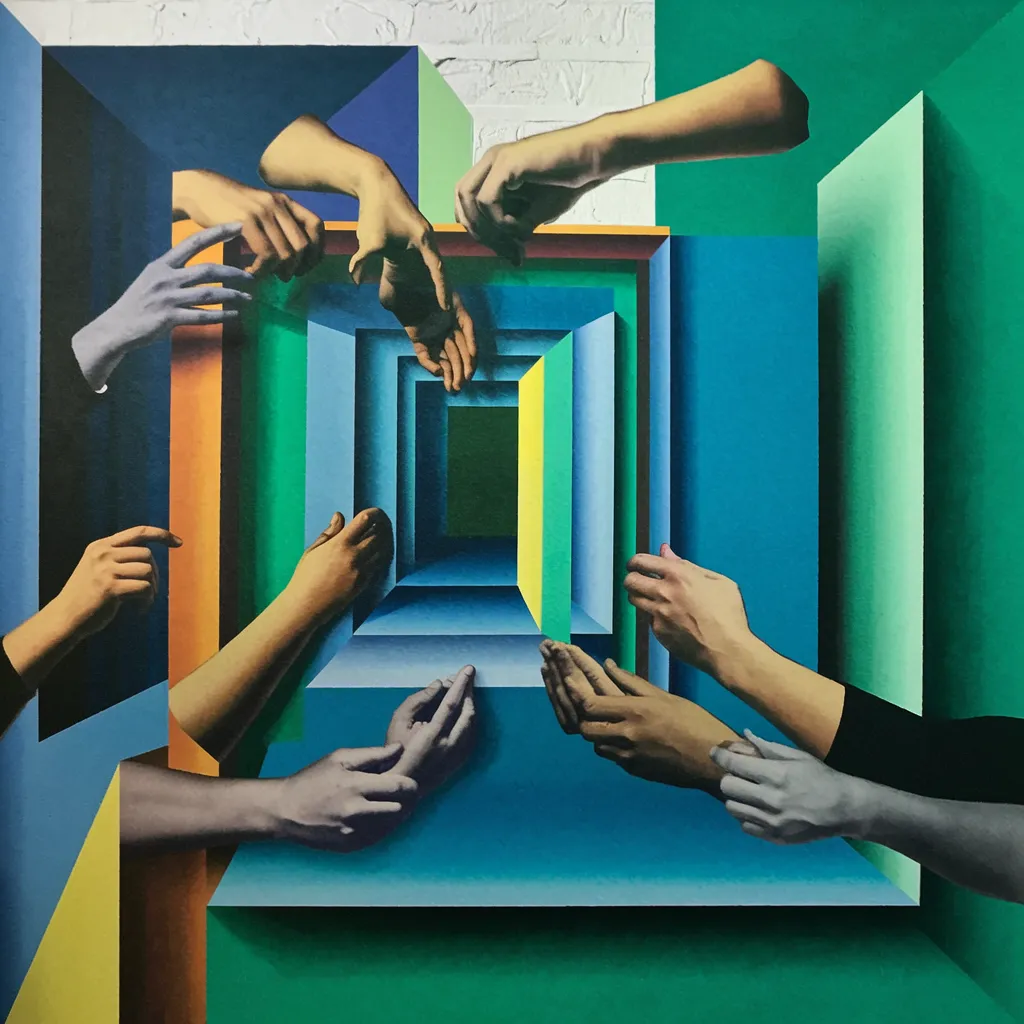
The ecosystem taxonomy can be seen below:
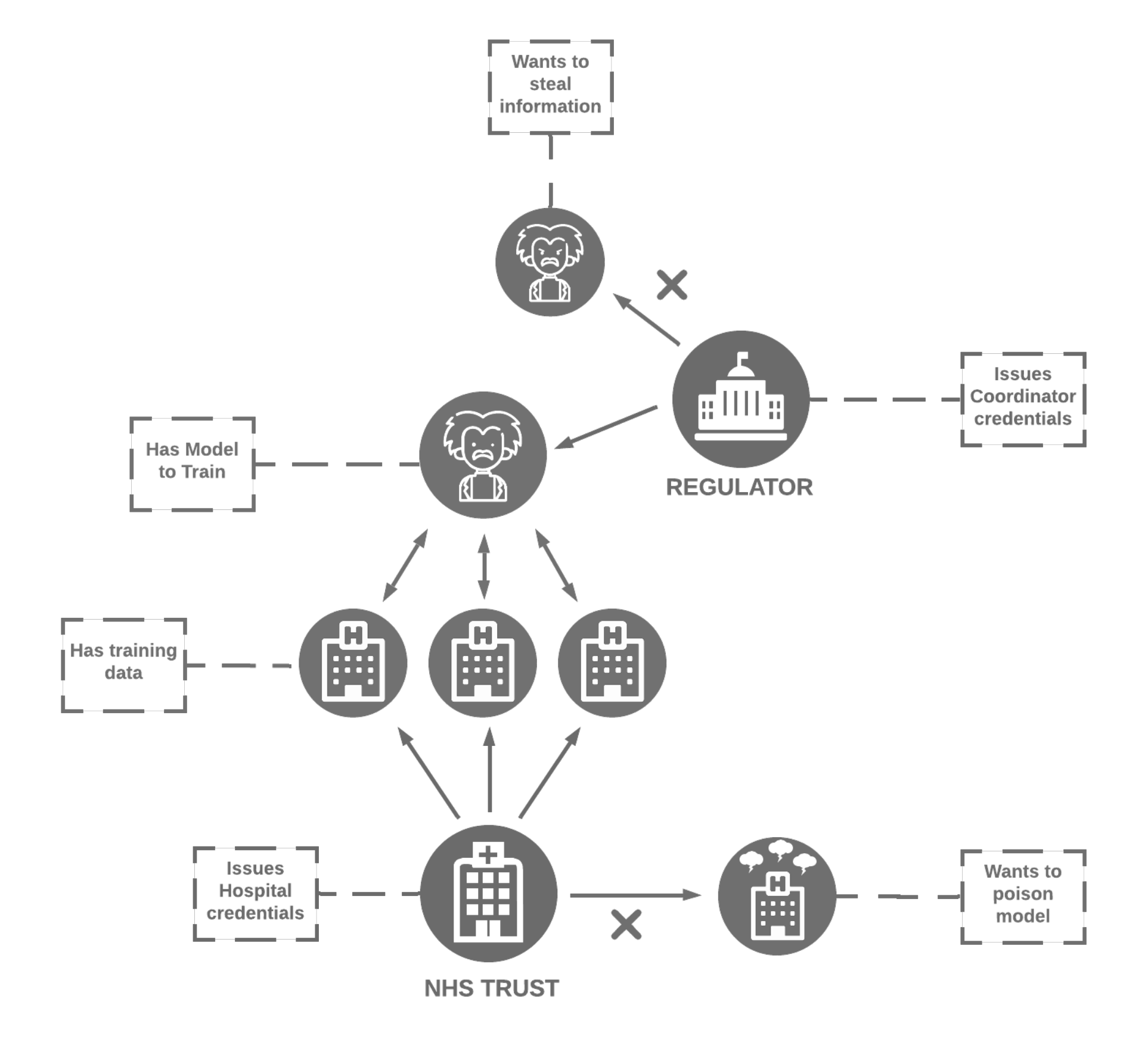
- Regulator
- Issues Coordinator Credentials.
- Ensures only authorized entities (e.g., hospitals, data scientists) participate in the federated learning process.
- Acts as a gatekeeper to block malicious actors (e.g., entities wanting to steal information).
- Coordinator
- Has a Model to Train and collaborates with hospitals to train the model on distributed data.
- Communicates with both the Regulator and Hospitals.
- Is verified by credentials issued by the Regulator.
- NHS Trust
- Acts as a middle layer managing hospitals within its network.
- Issues Hospital Credentials to authenticate participating hospitals.
- Ensures hospitals provide training data securely and blocks entities attempting to poison the model.
- Hospitals
- Possess Training Data for the federated learning model.
- Collaborate with the coordinator to securely train the model.
- Are authenticated via credentials issued by the NHS Trust.
5. Threat Actors
- Wants to Steal Information: Malicious actors aiming to extract sensitive information from the federated learning system. Blocked by the Regulators.
- Wants to Poison Model: Malicious actors aiming to corrupt the learning process by introducing poisoned data. Blocked by the NHS Trust.
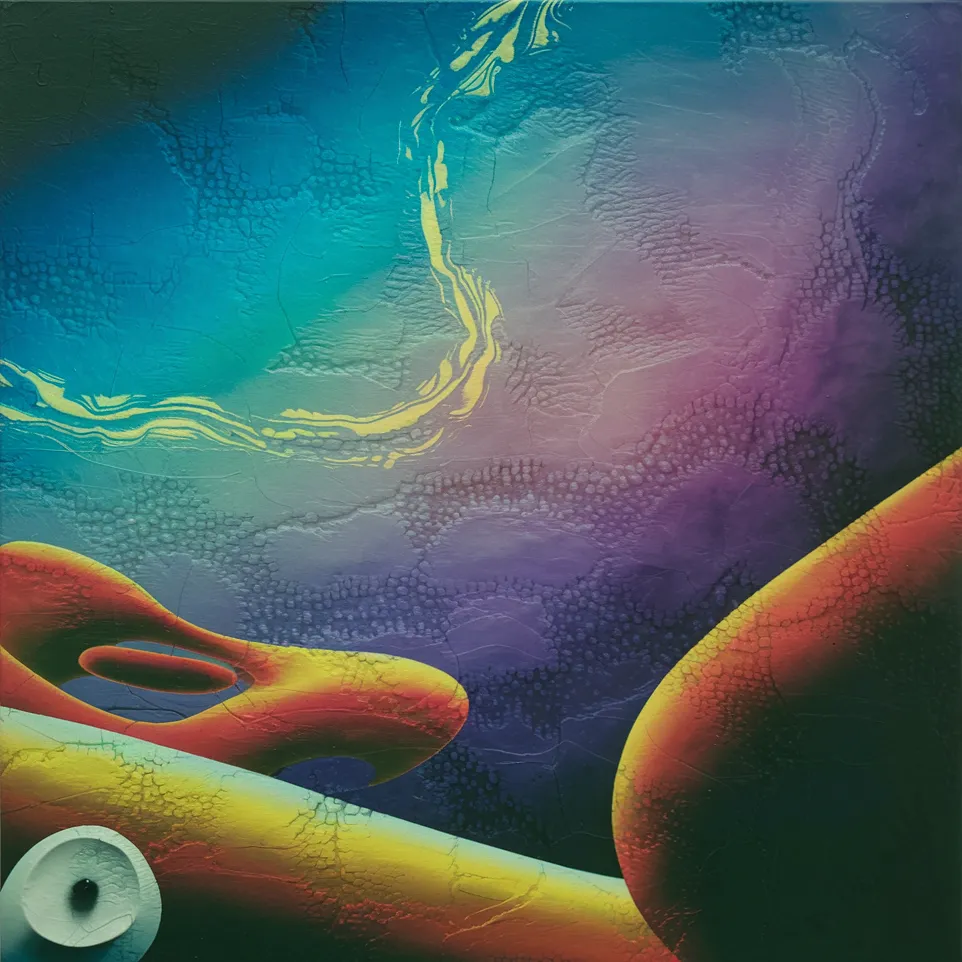
Document Source: Digital Trust Toolkit